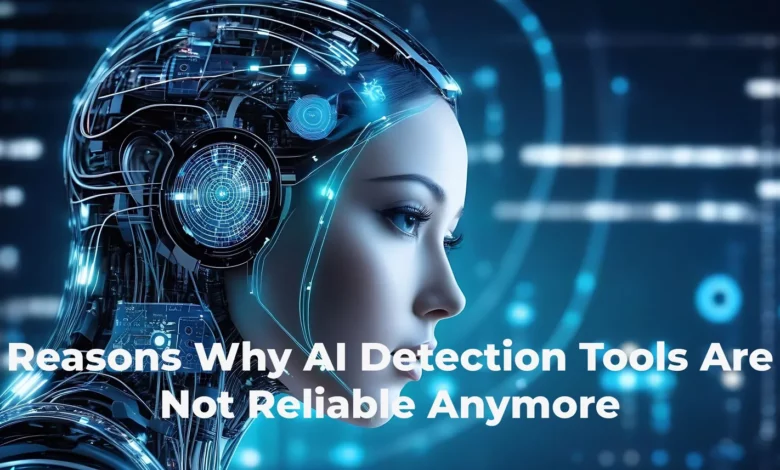
The AI revolution is happening everywhere, and history teaches us that whatever works better usually becomes the norm. Some folks, such as teachers and businesses, may want to stop AI entirely, but that’s really hard to do. This is because there are freely available tools, no strict rules, and it’s tough to enforce bans, especially when AI detectors might not be reliable enough for use in legal situations. So, what’s the smartest way to handle this situation?
It’s significant to realize that simulated AI based tools that check content may not necessarily get things right. The primary thing to recall isn’t to totally trust them. All things considered, utilize alternate ways of checking assuming the author of the best digital marketing institute in Delhi is familiar with the subject. Assuming that you’re uncertain, ask them inquiries connected with the substance to check whether they truly know what they’re talking about.
Ai Detection Tools Have Zero Accuracy
These tools conduct a thorough, sentence-by-sentence examination of academic papers, assigning scores based on the extent of AI involvement in the text. Their implementation in universities is seen as advantageous, potentially dissuading students from resorting to AI assistance. However, the reliability of these tools falls short of the ideal standard. The primary issue with AI detection tools lies in their elevated false positive rates.
This implies a tendency to misidentify human-authored content as AI-generated, even when no AI was utilized in its creation. Some AI detection companies, like Turnitin, assert a low 4% false positive rate. Despite the seemingly high accuracy suggested by this percentage, the implications are significant.
Bias in Training Data
AI models learn from data, and if the training data is biased, the model may inherit those biases. This can result in inaccurate predictions, especially for underrepresented groups. When these tools learn from datasets that reflect societal biases or imbalances, they can perpetuate and amplify those biases.
This bias can result in unfair and discriminatory outcomes, particularly for underrepresented groups. Recognizing and mitigating such biases is crucial to ensure the ethical and equitable deployment of AI systems across diverse populations and use cases.
Adversarial Attacks
AI systems can be vulnerable to adversarial attacks where small, carefully crafted changes to input data can cause the model to make significant errors. Adversarial attacks exploit vulnerabilities in AI systems by making imperceptible modifications to input data. These subtle changes, often undetectable to humans, can mislead the model into making significant errors.
For example, adding carefully crafted noise or perturbations to an image can cause an image recognition AI to misclassify the object it perceives. Adversarial attacks highlight the model’s sensitivity to minute alterations, posing a challenge in deploying AI systems, especially in security-critical applications, as they may not reliably withstand manipulations intended to deceive or compromise their accuracy.
Lack of Diversity in Testing Data
If the testing data used to evaluate the model’s performance does not reflect the diversity of real-world scenarios. The model may not generalize well to new, unseen situations. If the data used to evaluate these tools does not adequately represent the varied scenarios encountered in the real world, the model may struggle to generalize effectively.
This can lead to inaccurate predictions in novel situations, as the AI system may not have encountered diverse contexts during its training, hindering its ability to perform reliably across a broad range of scenarios and conditions.
Limited Context Understanding
AI models may struggle to comprehend context and nuance, leading to misinterpretation of complex situations or sarcasm, particularly in natural language processing tasks. These tools may struggle to grasp the intricacies of context and nuance within human communication. In natural language processing, the models might misinterpret sarcasm or fail to comprehend complex scenarios accurately.
This limitation arises from the inherent difficulty of teaching machines to understand the subtle and often culturally dependent aspects of human language, making it crucial to acknowledge the potential for misinterpretation and lack of contextual awareness in AI systems.
Dynamic and Evolving Threat Landscape
The rapid evolution of tactics used by malicious actors can outpace the ability of AI detection tools to adapt, making them less effective in identifying new and sophisticated threats. As threat landscapes constantly change, new and sophisticated techniques emerge, challenging the ability of these tools to keep pace.
The tools may struggle to identify and mitigate novel threats promptly, leading to a potential gap in their effectiveness. Continuous research and updates are essential to enhance the tools’ capabilities and address the evolving nature of cyber threats in order to maintain their reliability and relevance in real-world scenarios.
Overreliance on Pattern Recognition
AI models often rely on pattern recognition, and if the patterns in the data change, the model may become less accurate. This is particularly challenging in dynamic environments. As patterns in data constantly evolve, especially in dynamic environments, models may struggle to adapt quickly.
If the data patterns shift, the model’s effectiveness diminishes, leading to inaccuracies in predictions. This limitation poses a significant hurdle, particularly in areas where real-world conditions are subject to frequent changes, requiring continuous model updates to maintain reliability.
Data Poisoning
If attackers can manipulate the training data, they might introduce subtle changes that compromise the model’s integrity and cause it to make incorrect predictions. By strategically injecting misleading information during the training phase, attackers can influence the AI model to make incorrect predictions or exhibit biased behavior.
This undermines the system’s reliability, as it learns from corrupted data, potentially leading to inaccurate outcomes and diminishing the tool’s effectiveness in accurately detecting and responding to real-world scenarios. Preventing and detecting data poisoning attacks is crucial for maintaining the trustworthiness of AI detection tools.
Excessive False Positives or Negatives
AI detection tools may produce false positives (flagging non-threats) or false negatives (failing to detect actual threats), impacting the tool’s reliability and trustworthiness. High false positives can lead to unnecessary alerts and wasted resources, while false negatives pose serious risks by allowing genuine threats to go unnoticed.
Striking the right balance is crucial for the tool’s reliability, as an imbalance may erode user trust and hinder effective threat mitigation. Achieving optimal accuracy requires ongoing refinement and adjustment to minimize both types of errors in the detection process.
Lack of Explainability
Numerous AI based models, particularly profound learning models, work as complicated secret elements, making it trying to grasp the thinking behind their choices. Absence of interpretability can diminish trust in the apparatus’ dependability. Many models, particularly profound learning ones, work as mind boggling secret elements, making it challenging for people to figure out the reasoning behind their choices.
This lack of transparency can lead to reduced trust in the reliability of AI detection tools, as users may be unable to comprehend how and why a particular prediction or classification was made. Explainability is crucial for ensuring accountability, understanding potential biases, and gaining user confidence, especially in sensitive applications such as legal, medical, or security domains.
Conclusion
As more and more content created by artificial intelligence (AI) becomes popular, there are tools claiming to determine if a human or AI wrote something. But currently, the best digital marketing company in Delhi couldn’t find a tool that accurately identifies both AI-generated and human-written content. It’s like a competition between AI content creation and the tools trying to spot it. Right now, the tools are struggling to keep up because AI content generators, such as GPT-3 and GPT-4, keep getting better with new and improved algorithms.